News
Can AI play a role in weather warning systems?
By: Edwin Gilson
Last updated: Wednesday, 19 June 2024
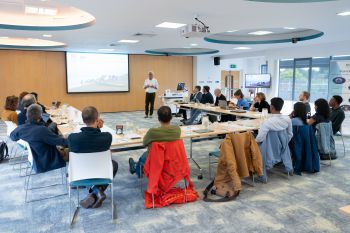
Professor Filippo Osella presents his research at the workshop
This question was at the heart of a workshop entitled ‘AI for Improving Weather Warning Systems’, hosted by the Sussex Sustainability Research Programme (SSRP), Sussex AI, and Sussex Digital Humanities Lab (SHL) on Thursday 13 June.
Researchers from the three Centres of Excellence discussed the potential of AI to forecast extreme events attributed to human-caused climate change, such as drought, heatwaves, cyclones, and flooding.
Introducing the workshop, SSRP Director Professor Joseph Alcamo pointed out that 85 percent of people affected by extreme weather events live in Africa and Asia, in communities that are least well equipped to cope with such disasters.
There will be an increase in these events over many parts of the world in the years and decades to come, Professor Alcamo added. This means we need to shield populations and buy time – which is where early warning systems come in.
Professor Alcamo described early warning systems as the ‘first line of defence against climate change’, but not the ultimate solution.
The purpose of this workshop, Professor Alcamo said, was to find out what work is currently being done on warning systems, to consider how AI could improve them, and assess the potential areas of collaboration between researchers from SSRP, Sussex AI, and SHL.
Global South case studies
Experiences from two major Sussex early weather warning projects were presented at the workshop. Firstly, SSRP Fellow Professor Filippo Osella shared findings from his SSRP-funded project on developing weather warnings for fisherfolk in the state of Kerala on the South West coast of India.
The ability to accurately track cyclones – where they might hit, and to what degree of intensity – is crucial to ensuring the safety and livelihoods of local fisherpeople in this region. Efficient, localised warning systems would also allow for more sustainable fishing, as the fishers can be more careful and targeted in their work.
Using a forecasting algorithm tool developed by American universities, Professor Osella and his collaborators have produced focused forecasts, covering three villages, which had a high level of accuracy. The team also asked fisherfolk about the weather conditions on a daily basis, which refined the data set.
The forecast strategy employed by Professor Filippo Osella and his research team in Kerala
Professor Osella added that communication is also key in the development of warning systems. To this end, his team have introduced numerous channels designed to inform local fishers of weather risk, from alert screens in the three villages to WhatsApp groups and even a mobile app.
Discussing the desired next steps of the project, Professor Osella said a comprehensive and efficient forecasting service was needed for the coastal communities.
AI could be used to hone the existing forecast technology, making it more effective and sensitive to data. Different forms of weather could be factored into the model, including rain, storms and waves as well as wind, and forecasts could be tailored according to the sizes of fishing boats.
Lastly, Professor Osella stressed the need for the forecasting to incorporate the experiences and knowledge of local people, becoming a ‘bottom up’ rather than ‘top down’ system. This message would recur throughout the workshop. Trust is key, Professor Osella concluded – we must involve fishers in the production of forecasts.
SSRP fellow Dr Pedram Rowhani then presented work he, Professor Martin Todd and collaborators have been carrying out on early warning systems for extreme weather events – and particularly drought – in the greater Horn of Africa.
He highlighted the importance of ‘anticipatory action’; the ability to predict rather than merely react to the impacts of drought. Ideally, AI would enable researchers to create reliable forecasts that communicate risk to local people and ensure contingency plans are set before drought takes hold.
Dr Rowhani’s collaborative project is entitled PASSAGE: strengthening PAStoral livelihoodS in the African Greater horn through Effective anticipatory action’. The project encompasses Somalia, Ethiopia, South Sudan, Kenya and Uganda. Pastoral farmers in this region cross national borders all the time as they move sheep and goats to various grazing areas.
The area covered in the PASSAGE project
Grass forage and water are two important resources that pastoral farmers depend on, and both are negatively affected by drought and aridity.
Regular rain patterns in the region have been disrupted by climate change, Dr Rowhani added, meaning provincial knowledge is potentially less reliable than it would once have been. Nonetheless, like Professor Osella, he emphasised the importance of foregrounding the experience of local workers.
Dr Rowhani said that he and his collaborators are trying to move from thinking about ‘what the weather will be’ to ‘what the weather will do’. It’s more important for people on the ground to understand what drought and rain will do, how it will affect the land and populations.
Artificial intelligence has the potential to improve data collection processes, including information on climate impacts and land use, Dr Rowhani added. As with Professor Osella’s work in South India, AI could streamline existing methods.
How AI can help
Next, Professor Julie Weeds gave a talk entitled ‘Natural Language Processing (NLP) for Weather Systems’. NLP is concerned with the processing of language and data – written or spoken – which would usually require human intelligence.
Typical NLP applications include classification, information extraction, machine translation, summarization and question answering (such as ChatGPT).
Professor Weeds stressed that there should be a clear reason for the use of NLP, and that AI is liable to errors, as we have seen with ChatGPT. However, she also highlighted the potential of the technology.
For instance, she posed the question of how NLP might automatically translate forecasts from official weather services into language that is clearer to the fisher folk and pastoral farmers at the centre of Professor Osella and Dr Rowhani’s projects.
Professor Weeds then provided some examples of how NLP might be applied. She used ChatGPT to translate a weather warning from English into Malayalam, the language primarily used in Kerala. Several of Professor Osella’s Indian collaborators who attended the workshop confirmed the translation was fairly accurate.
Professor Weeds then showed how AI can extract relevant information from, summarise, and simplify scientific text. AI can also translate data from tables and charts into accessible copy.
An example of Chat GPT's text simplification function presented by Professor Weeds
In a discussion following Professor Weeds’ talk, she added that the more certain languages appear on the internet, the better AI’s translation performance will be.
The final presentation of the afternoon, on ‘Machine Learning for Weather Warning Systems’, was given by Dr Ivor Simpson.
Machine learning can play an important role in these systems, Dr Simpson said, because it enables predictions of new data rather than simply analysing existing data. As Dr Rowhani argued in his presentation, we need to be proactive rather than reactive in anticipating extreme weather events.
The potential of machine learning lies in its capacity to model climate trends which could, in turn, inform weather warning systems.
Dr Simpson made the point that outliers are always hard to predict, and it is difficult to make statistical models that deal with them. It is challenging to predict (once) rare extreme weather events, but these events are becoming more common, which, while undesirable in many other ways, is statistically beneficial.
Dr Ivor Simpson's definition of Machine Learning
Dr Simpson also signposted the issue of confidence, which is important to quantify. How confident can we be about our predictions? This needs to be factored into the data models.
Like the other presenters, Dr Simpson also underlined the need to ‘bake local knowledge’ into machine learning models. Moreover, the trustworthiness of AI is always an issue, as emphasised in Professor Weeds’ presentation.
Conclusions
Drawing together the presentations and discussions conducted throughout the afternoon, Professor Alcamo presented some preliminary conclusions of the workshop:
AI has a range of applications that can potentially improve existing weather warning systems:
- AI can be used in existing weather warning systems as a kind of shortcut for low-income countries and marginalised communities who don’t have sufficient existing forecasting personnel and technology. For example, AI could be used to translate technical weather forecasts to the language of marginalised communities, bypassing the need to train and hire experienced experts to do this translation.
- AI can be used to identify the impact of weather and climate-related events (perhaps using data from X traffic or on-the-ground data collection).
- AI can be used to capture local knowledge and embed it in weather warning systems. For example, AI could be used to collect special knowledge of farmers and fisher about local weather patterns and the impacts of extreme weather events.
- AI can be used to process weather data for improving seasonal forecasts.
- AI can be used to articulate confidence intervals of weather forecasts.
- AI can be used to predict behaviour of decision making (but be alert to data-biases in AI applications).
Rather than using AI for the sake of it, participants agreed about the importance of defining the questions we want AI to answer, and the services we want it to provide.
As the beginning of a hopefully long and fruitful collaboration between SSRP, Sussex AI and SHL Digital on the subject of AI and weather warning systems, the workshop made excellent progress in tackling these complex and urgent issues.
Contact
Contact the School office: lps@sussex.ac.uk.